How Moving Beyond Real Time Optimisation Can Revolutionise Gaming
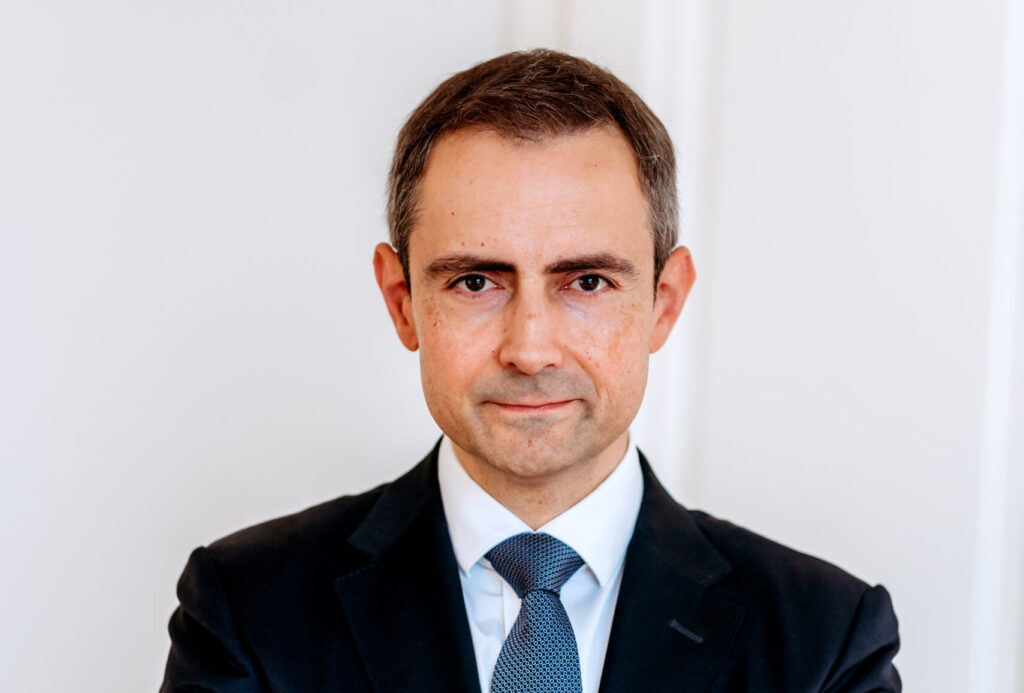
Acting in Advance – New Ways in Platform Operations
Given the giant strides forward the iGaming industry has made in terms of data acquisition, AI and machine learning in recent months, I believe we’re now entering a pivotal moment where the better-run platforms are switching from being reactive to proactive.
These days, more and more companies are starting to employ prediction-based models that are informed by reliable data to make educated guesses about how their customers are likely to behave – and that’s definitely a big deal for the sector.
Arguably the best way to think about these optimised predictions and how they can assist your business is to liken the process to having a very local weather forecast. When you have this information, you can better prepare yourself for the day ahead and utilising predictions is certainly no different – armed with reliable data that’s structured in a coherent manner, you can begin painting an accurate picture of user activity on your platform in general and also identify specific patterns in customer behaviour.
Let’s take an example where the information I’ve collected has identified an increased risk of churn building up during a session. Here, better data collection and modelling can now help me make an optimised prediction about what is most likely to happen within a certain corridor of probability. I can then, in turn, react to this information – particularly if there’s an opportunity to do so in real-time – the moment the customer is interacting with my product, rather than waiting for the churn to happen.
The action I could take here might be something like giving a bonus to a certain user or inviting them to come and play in a tournament, but whatever the engagement feature in your system offers, I’d be doing it based on a high-probability prediction before they’ve actually moved away from my product. This is one of the core effects that have an impact at bottom line and overall success, as reactivating a customer is always far more labour-intensive – not to mention expensive – than pre-empting their actions and engaging with them proactively to ensure this churn doesn’t occur.
Data science companies like Golden Whale are already starting to work with businesses to improve this aspect of their offerings, and I believe it’s an area where we can add great value to the industry. Even if an operator doesn’t choose to work with the tools and infrastructure a data science company provides, the most important thing is that they can help them get their data acquisition right – and whatever role they fill in the industry, this is the basis of the future goldmine they’re building towards.
Self-Optimising Game Systems with Reinforcement-Based Structures
Where I think things get even more interesting, however, is when machine learning and self-optimising systems also come into the fray. As the goal of a good self-optimising system is to continually ingest user data and adapt its models to make more accurate assumptions as player behaviour evolves over time, combining optimised predictions with machine learning creates some very exciting possibilities – and I believe this will ultimately herald the start of a major reinforcement cycle within the industry.
Essentially, we’ll soon present systems where the learnings companies have in their models are being fed back into the system in operation, with this resulting in self-inflicted changes to the data that then comes from this system back into their results. When done correctly, what we’ll then get is an iterative loop that not only enables companies to market changes effectively, but simultaneously improves the quality of its predictions – which, remember, can go right down to the user level – over the long term.
Of course, self-optimising systems aren’t able to “invent” actions by themselves, but what they can do is enable companies to come up with more ideas about how to react to certain user behaviours and quickly and iteratively check how they can optimise a feature so that it works even more effectively than the one that preceded it. This will steepen the learning curve and – once up and running – allow them to learn more about player behaviour in one year than they otherwise would have done in ten.
Considering the value optimised predictions based on solid data acquisition can add to a business – and how this value can be ratcheted up further when self-optimising systems are also added into the mix – I believe it’s fundamentally important that operators and developers begin exploring the possibilities these new technologies can provide on the basis of solid infrastructure. Once they make the switch, they can begin moving away from the cycle of constantly reacting to developments after they’ve happened and instead start proactively creating a more positive user experience that will only grow more sophisticated with time.
by Eberhard Dürrschmid, CEO at Golden Whale Productions
Eberhard founded and built up gaming heavyweight Greentube as a business and was the CEO from the start to the successful sale of the group. An investor and consultant in several tech businesses, Eberhard also has a PhD in Biotech with a focus on Data Science.